A blog post by Matthew Venne, Sr. Solutions Director, stackArmor
It’s no secret that Cloud 2.0 will be driven by Artificial Intelligence (AI). The rate at which the world is adopting AI-based solutions is nothing short of staggering; what was once viewed as science fiction, is quickly becoming science fact. With each AI system that gets deployed into the world, another machine takes ownership of decisions previously made by a human. We are currently seeing AI being adopted across all sectors of life: self-driving cars, college admissions, housing applications, the defense industry and more. This presents unique challenges and risks that require new thought leadership to properly assess and mitigate.
What happens if:
- An Autonomous Intelligence System (AIS) used for college admissions incorporates a bias against any demographic?
- An AI-powered smartwatch discovers its owner has a condition they wanted to keep private?
- An AIS escalates its own privileges to access unauthorized systems and/or services?
The risk and implications for government agencies and public sector agencies responsible for regulating healthcare, education and wide sections of the US economy are profound. To help answer these questions for organizations especially in highly regulated markets, stackArmor has focused on developing a standards-based governance model that is rooted in National Institute of Standards & Technologies (NIST) frameworks including AI Risk Management Framework (RMF), NIST RMF and NIST SP 800-53. NIST AI RMF provides a starting point for understanding risk vectors and how to go about risk mitigations. We believe that training and equipping existing IT and Cybersecurity risk assessors with additional understanding of AI specific challenges like safety, bias and explainability will accelerate safe adoption of AI systems. However, there aren’t many standards to go by for helping train the next generation of AI-smart assessors and risk management professionals. Fortunately, we found a course from Institute of Electrical and Electronics Engineers (IEEE) to become a CertifAIEdTM Ethical AI Assessor.
In this blog we outline our experiences with the IEEE CertifAIEdTM program and how FedRAMP, FISMA and Cybersecurity/IT systems risk management professional can evolve their skills to be relevant in an AI-powered world.
What is the IEEE CertifAIEdTM?
In the IEEE’s own words:
IEEE CertifAIEdTM is a certification program for assessing ethics of AIS to help protect, differentiate, and grow product adoption. The resulting certificate and mark demonstrates the organization’s effort to deliver a solution with a more trustworthy AIS experience to their users.
Through certification guidance, assessment and independent verification, IEEE CertifAIEd offers the ability to scale responsible innovation implementations, thereby helping to increase the quality of AIS, the associated trust with key stakeholders, and realizing associated benefits.
An IEEE CertifAIEdTM assessment is framed around 4 Core Ontologies:
- Transparency –An AIS must be fully open and honest about its processes. This includes mechanisms to explain how each AI decision was made and the confidence in the system’s performance through training and testing. One way to look at it: can a user reasonably expect the AIS to perform in a predictable manner and what is the evidence of this?
- Accountability – An AIS must take ownership of its decision-making process – both technically and organizationally. For example, if the AIS returns a potentially damaging result – what mechanisms does the organization have in place for mitigation? Who is responsible? Does the user have ability to refute the result? Is there a human-in-the-loop to oversee the training, testing, and actual results?
- Privacy –Mechanisms must be in place to protect the user and the data they provide to the AIS. This includes user anonymity and confidentiality. What happens to a user’s data when they stop using the AIS?
- Algorithmic Bias –Processes and policies should be implemented around the use of protected characteristics to ensure they treat all users fairly – regardless of gender, religion, race, sexual preference, etc. An organization may be required to demonstrate their existing policies that show why the use of the characteristics is required in the model and how they help the organization achieve their desired goals. From a technical perspective, organizations may be required to demonstrate the mechanisms used to ensure that each protected characteristic is not over/or undervalued during tuning, training, and testing of the AIS.
How do IEEE CertifiAIEdTM assessments run?
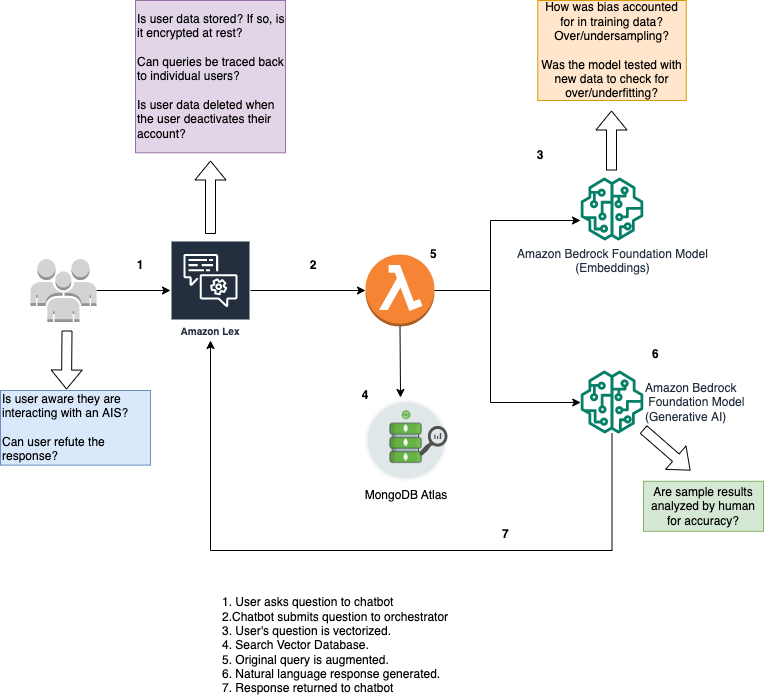
Figure 1: Here is a theoretical chatbot that a utility company uses to respond to user requests. The chatbot is powered by AWS Bedrock and leverages Retrieval Augmented Generation. Several ethical questions about the system are called out.
Assessments are performed by teams of CertifAIEdTM Assessors lead by a CertifAIEdTM Lead Assessor, which is an official designation given to the most experienced and adept assessors. Ideally, the assessment teams are comprised of assessors with a wide range of backgrounds and experience.
Each of the four ontologies has its own list of drivers and inhibitors, which are specific criteria that must be accounted for and documented during the assessment.
- Drivers – Demonstrate how the AIS strives towards the embodiment of the ontology – the more evidence of drivers the better.
- Inhibitors – Demonstrate how the AIS does NOT embody the ontology – the less evidence of inhibitors the better.
The number of drivers and inhibitors required for the assessment is dependent on the level of impact an AIS could have on the given ontology. It is the job of the assessor to determine the level of impact based on the likelihood of an AIS impacting the ontology and the severity that impact will have
The following is an example of a driver under the Transparency ontology:
Organizational governance, capability, and maturity – This comprises the capability, maturity, and intent of the development organization in having the right motivation and resources, processes, and so forth to achieve transparency.
Here is an example of an inhibitor under the Transparency ontology:
Concern with liability – The service provider’s awareness of potential risk exposure, and delivery of bare minimum (or inadequate) information to manage the risk. This could include legal, commercial, financial, and human intervention dimensions.
Additionally, each driver and inhibitor have Ethical Foundational Requirements (EFRs) which are individual evidentiary requirements that must be provided to the assessor. IEEE provides several EFRs for each driver/inhibitor by default to frame the assessment properly; however, the assessor does have the ability to customize the EFRs for each AIS which helps frame the discussion and assessment around the individual system.
Each EFR is then mapped to the Duty Holder responsible for providing the evidence:
- Developer – the person(s) who developed and designed the AIS.
- Integrator – the person(s) who deployed/integrated the AIS.
- Operator – the person(s) responsible for day-to-day operations of the AIS.
- Maintainer – the person(s) responsible for monitoring behavior of the AIS, performing system and model updates etc.
- Regulator – the person(s) responsible for enforcing laws, standards, and regulations that are relevant to the AIS.
Some EFRs may require input from all duty holders or a subset. It is possible that one person or group of people acts as multiple duty holders, depending on the implementation. This helps ensure that the proper entity is tasked with providing the evidence.
Once the assessment is complete, a report is submitted to the owner of the AIS being assessed and to an IEEE CertifAIEdTM Certifier. The certifier, which is a separate entity, reads the assessment and the recommendation of the assessor and makes a final decision on whether the AIS meets the ethical requirements of the ontologies.
The CertifAIEdTM program was first finalized in 2021 and there is a public blog regarding the first ethical assessment of an email classification system used by the City of Vienna, Austria.
My experiences with the course
I just missed the opportunity to enroll in a course in a US time zone. The next one was in Singapore time – 13 hours ahead of Eastern Time! I was hungry for knowledge, so I enrolled in the course anyways. The course ran 4 days (nights?) in a row from 8PM-4AM.– During that time, plenty of caffeine was consumed, I assure you, as I was still working my regular day job during the week. All the other enrollees were overseas, mostly Australia, China, and Europe. The course itself was hosted on Zoom and was both instructor-led and interactive. The instructors were CertifAIEdTM Lead Assessors, very friendly, and knowledgeable regarding the process.
Day 1 – The instructors outlined the overall CertifAIEdTM program and the importance of ethical assessment of AIS. There was a high-level discussion on the 4 ontologies, concluding with a workshop where the students broke up into groups and discussed how they would assess a theoretical AIS. Each group presented their thought process to the others and the trainers orchestrated a discussion. A big theme the trainers kept coming back to was “Why?”. Why did you choose this phrase? Why did you map it to this duty holder? Why did you customize this EFR? Why did you give the system a High impact?
Day 2 – Took a deep dive into Transparency and Accountability. Transparency is the most foundational of the ontologies, according to IEEE; with the other three ontologies having been derived from it. After reviewing each ontology in detail, we again separated into groups to assess another AIS through the Transparency and Accountability lenses respectively.
Day 3 – Retained the same deep dive and team review structure of Day 2 but, focused on Privacy and Algorithmic Bias.
Day 4 – Focused on the final exam,, which I am glad to say I passed! What I appreciated most about the final exam was that it was not a regurgitation of facts, but rather focused on demonstrating what my thought process would be as a CertifAIEdTM Assessor evaluating and AIS.
What’s next?
For me, the next step is to submit an application to IEEE to become an official Assessor. Once my application is evaluated, I would then be interviewed by a panel of CertifAIEdTM assessors who will consider my final exam results, interview responses and application to determine if I am qualified to become an IEEE CertifAIEdTM Assessor. Whether or not I am formally accepted as an assessor, this was one of the most fulfilling experiences of my career and it excites me to be better equipped for an AI-driven world.
As a senior technologist and solutions architect responsible for designing and implementing solutions that comply with FedRAMP, FISMA, DOD and CMMC 2.0 standards amongst others, it is critical to understand and incorporate secure and safe design principles right from the beginning. By understanding how risk assessments are performed, I can incorporate a lot of these learnings and perspectives into core systems design and implementation into a governance model we at stackArmor call ATO for AITM.